Data-Driven Models for Mixtures of Stereoselective Catalysts
The CATALOOP network aims at the development of powerful workflows for data-driven development of stereoselective catalysis. To achieve that, we will utilize comprehensive data-driven experimental approaches for realizing challenging asymmetric catalytic methods. This network brings together academic research groups with expertise in experimental catalyst development with theoretical groups skilled in computational and data-driven chemistry in order to develop new catalytic asymmetric reactions. World-leading industrial partners with a wide range of interests will provide advice on which approaches may have the most impact on industry and will host the students in secondments.
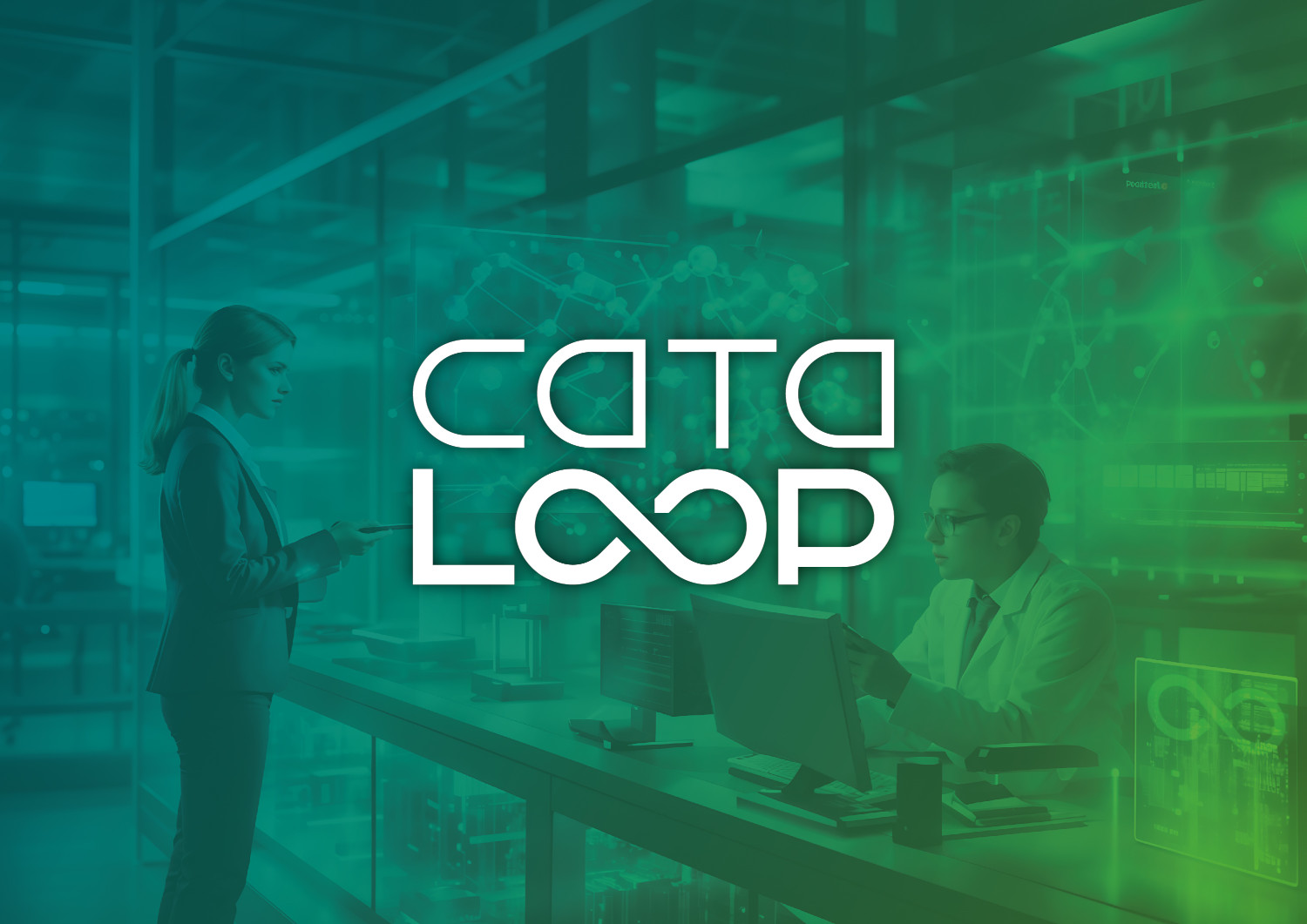
Position Details
Position: PhD Student
Start Date: October 2025
Chiral bidentate ligands belong to the most widely used co-catalysts in asymmetric catalysis. However, the synthesis of systematic libraries can be laborious, rendering data-driven bidentate ligand design challenging. In principle, one molecule of a bidentate ligand can be replaced with two molecules of monodentate ligands, enabling the use of combinatorial mixtures, which has been shown to lead to highly selective methodologies. This approach greatly simplifies the synthetic accessibility of viable ligand systems, but it leads to a combinatorial explosion of catalyst candidates. In this project, we will develop predictive data-driven models describing the catalytic activity and stereoinduction capabilities of ligand mixtures, allowing for their efficient experimental optimization. To apply, please submit your application material through the platform linked below.
Apply