Artificial Design
In recent years, artificial intelligence has achieved superhuman ability in playing the board game Go, and the popular video games StarCraft II and Gran Turismo 7. Additionally, state-of-the-art machine learning methods for protein structure prediction are currently revolutionizing biochemistry. Similarly, computer algorithms capable to design molecules with specific target properties are seeing an increasing implementation in both drug and material discovery. Our group develops, adapts and refines state of the art machine learning models for molecular design, so-called molecular generative models, to tackle challenging problems in homogeneous catalysis. Using the wealth of data that we collect during our data-driven catalyst optimization campaigns, our group implements these molecular design algorithms in our workflows to create new molecular catalysts.
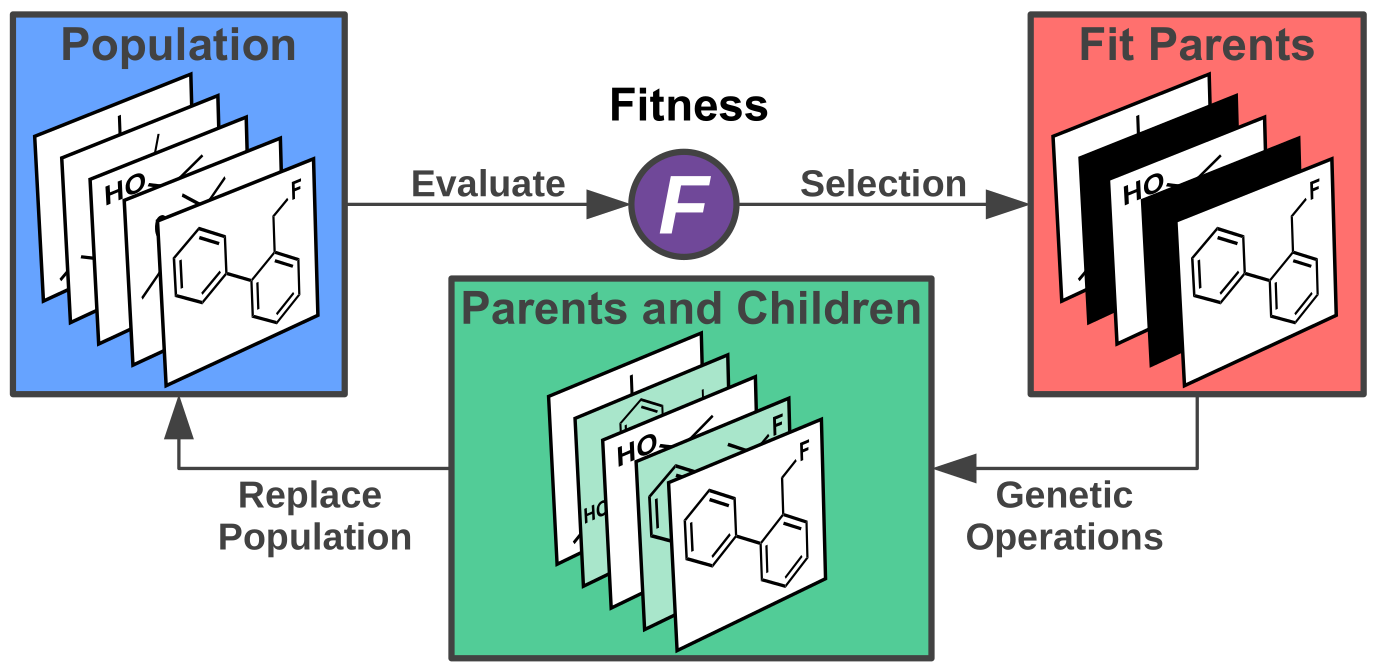
(Compare: https://doi.org/10.1021/acs.accounts.0c00785)
Molecular Generative Models
Among the wide range of available models for molecular design, evolutionary algorithms stand out because of their simplicity and their ease of use without requiring a significant amount of initial data. Accordingly, they are standard baseline models for inverse molecular design models, in particular for goal-directed optimization tasks. They can be the stepping stone for more sophisticated approaches such as reinforcement learning, which has provided ground-breaking advances in the field of artificial intelligence in recent years when fed with very large amounts of data. We seek to combine the best of both these worlds by implementing inverse molecular design algorithms that do not require any initial data to be started, however, at the same time, continuously learn from previous results to reduce the number of property evaluations significantly. This is important for real world applications in the laboratory as going beyond hundreds of experimental evaluations for catalytic activity tends to prove highly prohibitive.
Selected Publications
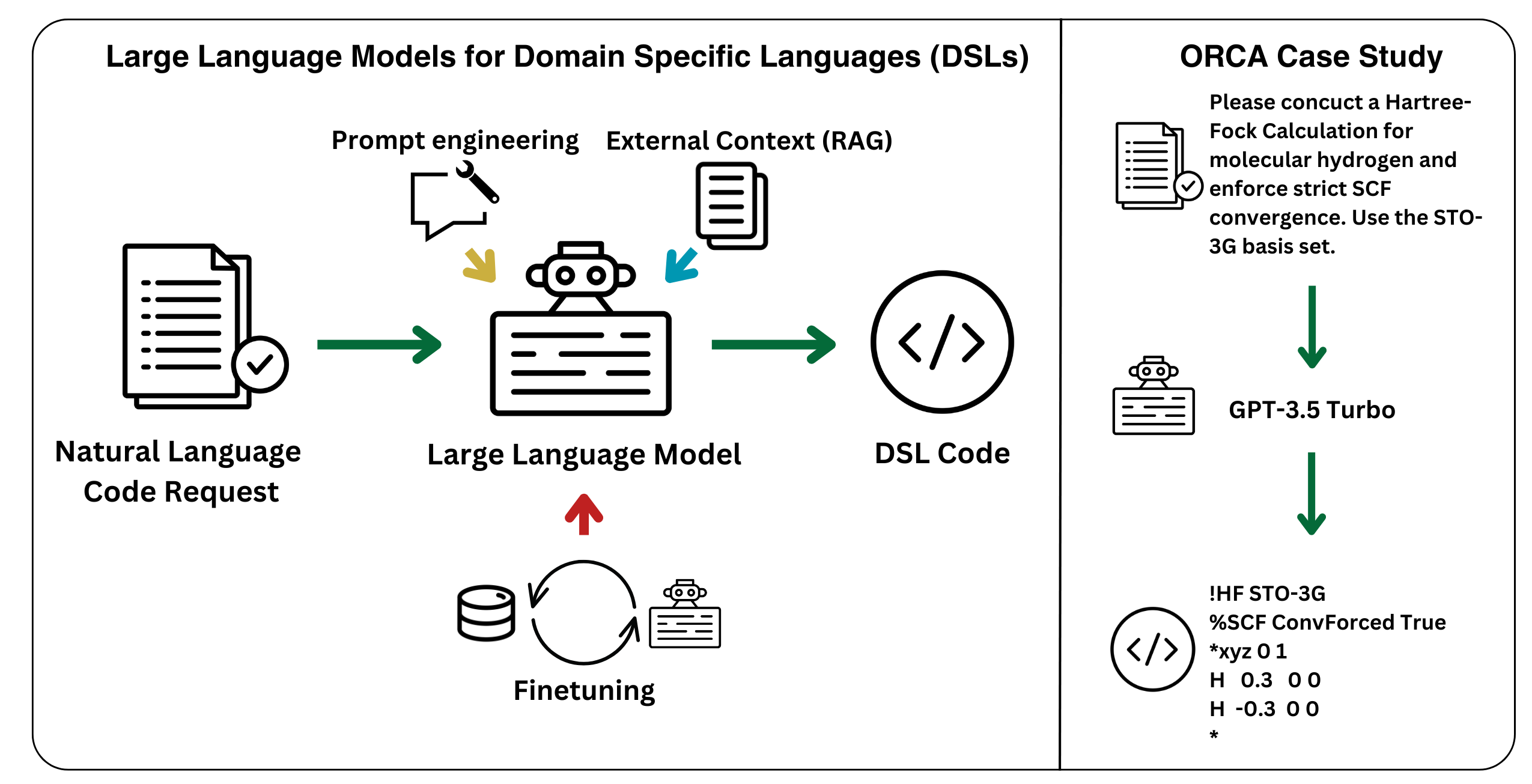
February 5, 2025
P. F. Jacobs, R. Pollice*
Digit. Discov. 2025, 4, 762.
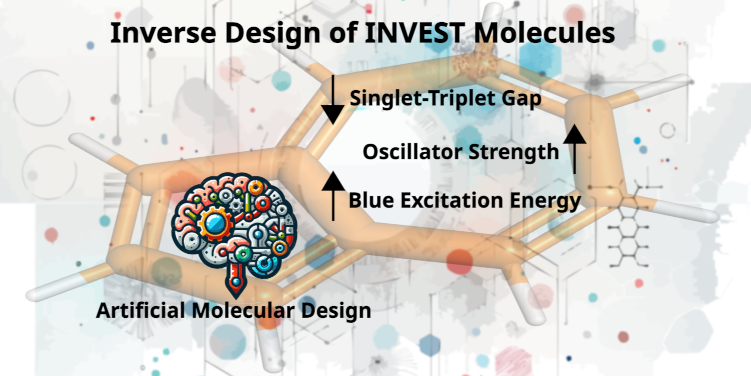
January 11, 2024
A. Nigam#, R. Pollice#,*, P. Friederich, A. Aspuru-Guzik*
Chem. Sci. 2024, 15, 2618.
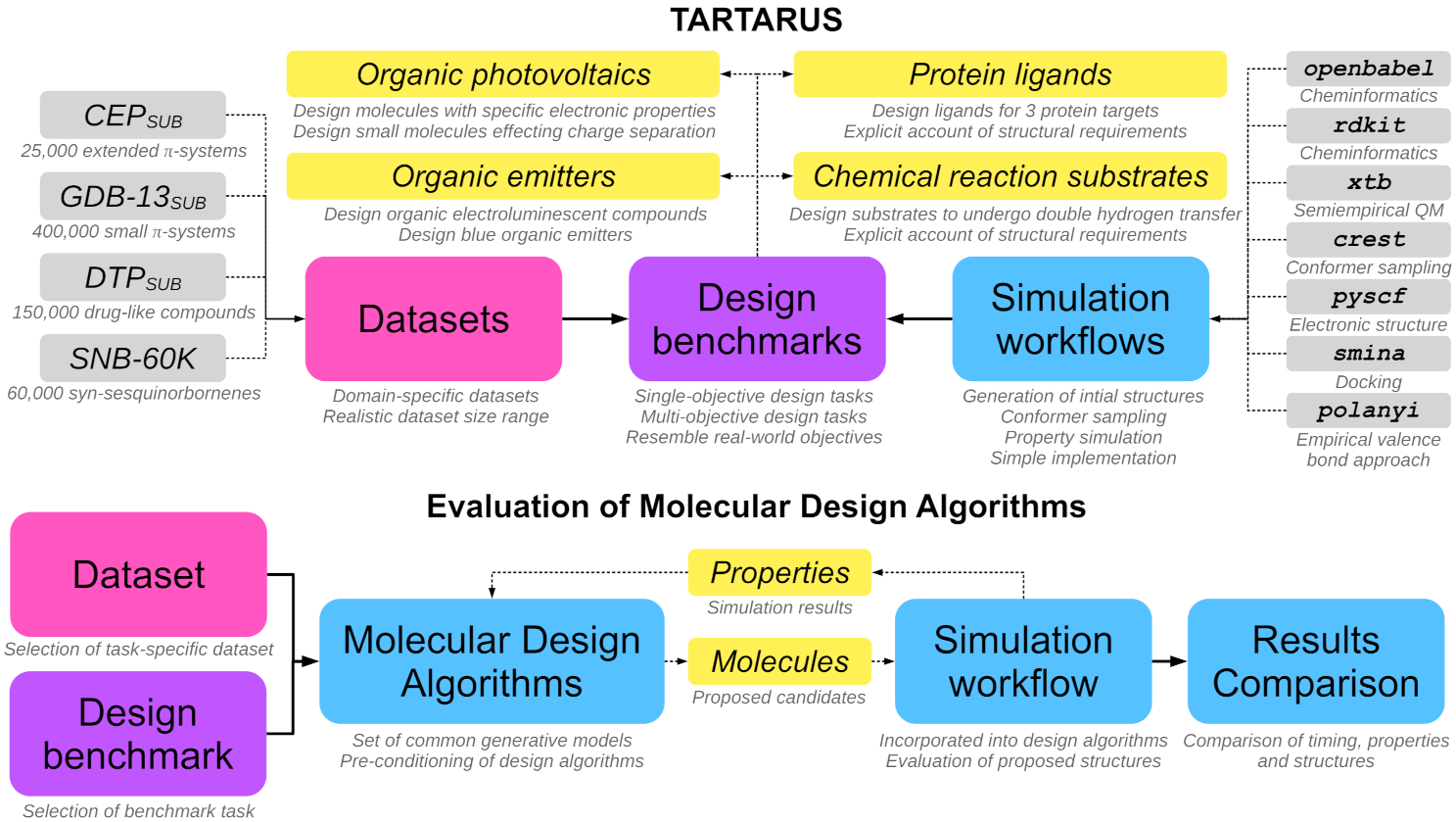
September 22, 2023
A. Nigam, R. Pollice*, G. Tom, K. Jorner, J. Wiles, L. A. Thiede, A. Kundaje, A. Aspuru-Guzik
Advances in Neural Information Processing Systems 2023, 36, 3263.